Automated Detection of Focal Cortical Dysplasias at GOSH using a surface-based approach
Focal cortical dysplasia (FCD) is a congenital abnormality of cortical development and a leading cause of surgically remediable drug resistant epilepsy. MRI has played a major role in the evaluation of patients; yet, significant proportions of lesions remain undetected by conventional image analysis. Machine learning offers a powerful framework to develop automated and individualised clinical tools that may improve the detection of lesions and prediction of clinically relevant outcome.
In work published in Neuroimage: Clinical in 2017, Adler, Wagstyl et al., developed a classifier using surface-based features to identify focal abnormalities of cortical development in a paediatric cohort from Great Ormond Street Hospital. Focal cortical dysplasias in this paediatric cohort were correctly identified in 73% of the children.
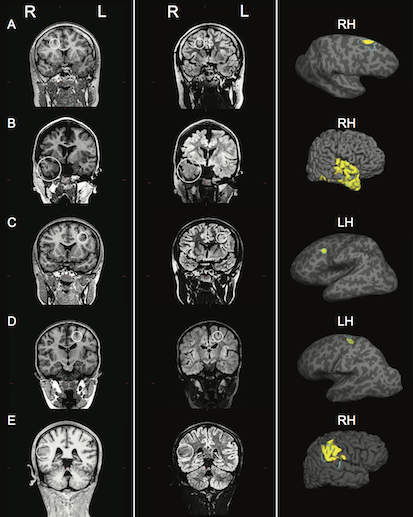
Further studies have since validated validated this method: Jin et al., 2018, in Epilepsia, Mo et al., 2018, Frontiers in Neuroscience, and Wagstyl, Adler et al., Epilepsia